Shuaifeng Zhi
I am now a Lecturer (Assistant Professor) at the Department of Electronic Science and Technology, National University of Defense Technology (NUDT), China.
Prior to being an academic scholar, I obtained my Ph.D degree at The Dyson Robotics Lab at Imperial College,
supervised by Prof. Andrew J. Davison and Dr. Stefan Leutenegger;
finished my MSc.Eng and B.Eng in NUDT, China.
My research interest lies in semantic SLAM and semantic neural representations, combining semantics and SLAM systems using learning-based approaches.
Email  / 
Google Scholar  / 
LinkedIn  / 
Twitter
|
|
Event and News
July 2023: Happy to announce that our paper PlaneRecTR got accepted to ICCV 2023, RaNeRF got accepted to T-GRS 2023!
June 2023: Happy to announce that our paper TsCM got accepted to T-PAMI 2023!
Jan 2023: Happy to announce that our paper EDAL got accepted to ICLR 2023!
Dec 2022: Happy to announce that our paper iLabel got accepted to RA-L!
Feb 2022: The codes and data of Semantic-NeRF are now released!
Jan 2022: Happy to announce that our paper ReCo got accepted to ICLR 2022.
Oct 2021: Attended GAMES Webminar of semantic scene representations.
Oct 2021: Attended ICCV 2021 3DReps Workshop and presented Semantic-NeRF in poster session.
Sep 2021: Gave a talk of Semantic-NeRF in a Webinar of neural implicit representations held by GAUSSIAN ROBOTICS and TechBeat.
July 2021: Happy to announce that our paper Semantic-NeRF got accepted to ICCV 2021 as Oral Presentation (top 3%)!
Feb 2019: SceneCode got accepted to CVPR 2019.
July 2018: Participated International Computer Vision Summer School (ICVSS 2018) in Sicily, Italy.
|
|
SSR-2D: Semantic 3D Scene Reconstruction from 2D Images
Junwen Huang, Alexey Artemov, Yujin Chen, Shuaifeng Zhi, Kai Xu, Matthias Nießner
IEEE Transactions on Pattern Analysis and Machine Intelligence (T-PAMI), 2024
arxiv
We leverage differential rendering to learn complete 3D scans with semantic labelling without any 3D annotations.
|
|
OS3Flow: Optical and SAR Image Registration Using Symmetry-Guided Semi-Dense Optical Flow
Zixuan Sun, Shuaifeng Zhi, Kai Huo, Xuecong Liu, Weidong Jiang, Yongxiang Liu
IEEE Geoscience and Remote Sensing Letters (GRSL), 2024
We introduce OS3Flow, a novel registration framework using implicit symmetry between heterogeneous SAR image pairs to extract high-quality semi-dense flow estimations.
|
|
Enhancing Information Maximization with Distance-Aware Contrastive Learning for Source-Free Cross-Domain Few-Shot Learning
Huali Xu, Li Liu, Shuaifeng Zhi, Shaojing Fu, Zhuo Su, Ming-Ming Cheng, Yongxiang Liu
IEEE Transactions on Image Processing (T-IP), 2024
arxiv /
IEEE Link
We explore the Source-Free Cross-Domain Few-Shot Learning (SF-CDFSL) problem, leveraging on an enhanced information maximization (IM) strategy and a transductive mechanism.
|
|
Looking Beneath More: A Sequence-based Localizing Ground Penetrating Radar Framework
Pengyu Zhang, Shuaifeng Zhi, Yuelin Yuan, Beizhen Bi, Qin Xin, Xiaotao Huang, Liang Shen
IEEE International Conference on Robotics and Automation (ICRA), 2024
We propose a sequence-based framework for Localizing Ground Penetrating Radar (LGPR) to address the challenges caused by
the extreme scarcity of underground features and their introduced false candidate matches.
|
|
PlaneRecTR: Unified Query learning for 3D Plane Recovery from a Single View
Jingjia Shi, Shuaifeng Zhi, Kai Xu
International Conference on Computer Vision (ICCV), 2023
arxiv /
video /
video (bilibili) /
code
PlaneRecTR is a vision transformer architecture with query-based learning, and for the first time unifies all subtasks of single-view plane recovery with a single compact model.
Mutual benefits between planar geometry and segmentation can be obtained by PlaneRecTR, achieving SOTA performance on ScanNet and NYUv2-Plane datasets.
|
|
RaNeRF: Neural 3D Reconstruction of Space Targets from ISAR Image Sequences
Afei Liu, Shuanghui Zhang, Chi Zhang, Shuaifeng Zhi, Xiang Li
IEEE Transactions on Geoscience and Remote Sensing (T-GRS), 2023
RaNeRF is a novel 3D target reconstruction method using only observed ISAR image sequences, built upon powerful neural radiance fileds.
|
|
Unbiased Scene Graph Generation via Two-stage Causal Modeling
Shuzhou Sun, Shuaifeng Zhi, Qing Liao, Janne Janne Heikkila, Li Liu
IEEE Transactions on Pattern Analysis and Machine Intelligence (T-PAMI), 2023
arxiv /
Early Access
We propose Two-stage Causal Modeling (TsCM) for unbiased SGG predictions. Taking the long-tailed distribution and semantic confusion
as confounders to the Structural Causal Model (SCM), we decouple the causal intervention into two stages. As a model agnostic method, TsCM
achieves a better tradeoff between head and tail relationships.
|
|
ROFusion: Efficient Object Detection using Hybrid Point-wise Radar-Optical Fusion
Liu Liu, Shuaifeng Zhi, Zhenhua Du, Li Liu, Xinyu Zhang, Kai Huo, Weidong Jiang
International Conference on Artificial Neural Networks (ICANN), 2023
arxiv
We propose ROFusion, a hybrid point-wise Radar-Optical fusion approach for object detection in autonomous driving scenarios, motivated by
dense contextual information from both the range-doppler spectrum and images.
|
|
Evidential Uncertainty and Diversity Guided Active Learning for Scene Graph Generation
Shuzhou Sun, Shuaifeng Zhi, Janne Heikkila, Li Liu
International Conference on Learning Representations (ICLR), 2023
OpenReview
We propose EDAL, a novel AL framework tailored for the SGG task leveraging Evidential Deep Learning (EDL) and diversity-based debias modules.
|
|
iLabel: Revealing Objects in Neural Fields
Shuaifeng Zhi*, Edgar Sucar*, Andre Mouton, Iain Haughton,
Tristan Laidlow, Andrew J. Davison
( * denotes equal contributions.)
IEEE Robotics and Automation Letters (RA-L)
arxiv /
video /
project page
We build an online interactive 3D scene labelling and understanding system upon 3D neural field representation of geometry, colour and semantics.
|
|
Bootstrapping Semantic Segmentation with Regional Contrast
Shikun Liu, Shuaifeng Zhi, Edward Johns, Andrew J. Davison
International Conference on Learning Representations (ICLR), 2022
arxiv /
code /
project page
We present ReCo, a new contrastive learning framework designed at a regional level to assist learning in semantic segmentation.
ReCo performs (semi-)supervised pixel-level contrastive learning on a sparse set of hard negative pixels.
With minimal extra memory footprint,
Reco boosts exsiting baselines by a large margin, revealing hierarchical similarities of various semantic classes as well.
|
|
In-Place Scene Labelling and Understanding with Implicit Scene Representation
Shuaifeng Zhi, Tristan Laidlow, Stefan Leutenegger, Andrew J. Davison
International Conference on Computer Vision (ICCV), 2021
(Oral Presentation)
arxiv /
video /
video (bilibili) /
project page /
code
We show that neural radiance fileds (NeRF) contains strong priors for scene cluster and segmentation. The internal multi-view consistency and smoothness make the training process itself a multi-view semantic fusion process.
Such a scene-specific implcit semantic representation can be efficiently learned with various sparse or noisy annotations, leading to accurate dense labelling of the full scene.
|
|
SceneCode: Monocular Dense Semantic Reconstruction using Learned Encoded Scene Representations
Shuaifeng Zhi, Michael Bloesch, Stefan Leutenegger, Andrew J. Davison
IEEE Conference on Computer Vision and Pattern Recognition (CVPR), 2019
arxiv /
video
We show that an efficient code representation is able to control the semantic label prediction of an image.
Latent codes of overlapping images can be jointly optimised to perform coherent semantic fusion.
We also show how this approach can be used within a monocular keyframe based semantic
mapping system where a similar code approach is used for geometry.
|
|
Toward real-time 3D object recognition: A lightweight volumetric CNN framework using multitask learning
Shuaifeng Zhi, Yongxiang Liu, Xiang Li, Yulan Guo
Computers & Graphics, 2017
bibtex
We propose LightNet, a light-weight 3D volumetric CNN for real-time 3D object classification.
This paper subsumes the 3DOR 2017 paper LightNet.
|
|
LightNet: A Lightweight 3D Convolutional Neural Network for Real-Time 3D Object Recognition
Shuaifeng Zhi, Yongxiang Liu, Xiang Li, Yulan Guo
Eurographics Workshop on 3D Object Retrieval (3DOR), 2017
bibtex
|
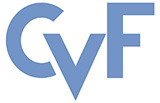 |
Reviewer in 2022: CVPR, ICLR, ICML, ICME, ICVR (ordinary PC member), WCCI
Reviewer in 2021: CVPR, ICCV, NeurIPS, ICLR, ICME, IJCNN
Reviewer in 2020: ICME
Reviewer in 2019: CVPR-W, ICCV-W, ICRA-W
|
 |
Lab Assistant, Robotics (Online with Coppeliasim!), Spring 2021
Lab Assistant, Robotics , Autumn 2019
Lab Assistant, Robotics , Spring 2019
Lab Assistant, Robotics , Autumn 2018
Lab Assistant, Advanced-Robotics, Spring 2018
|
Thank Dr. Jon Barron for sharing the source code of the website.
|
|